What is cluster analysis? A complete guide
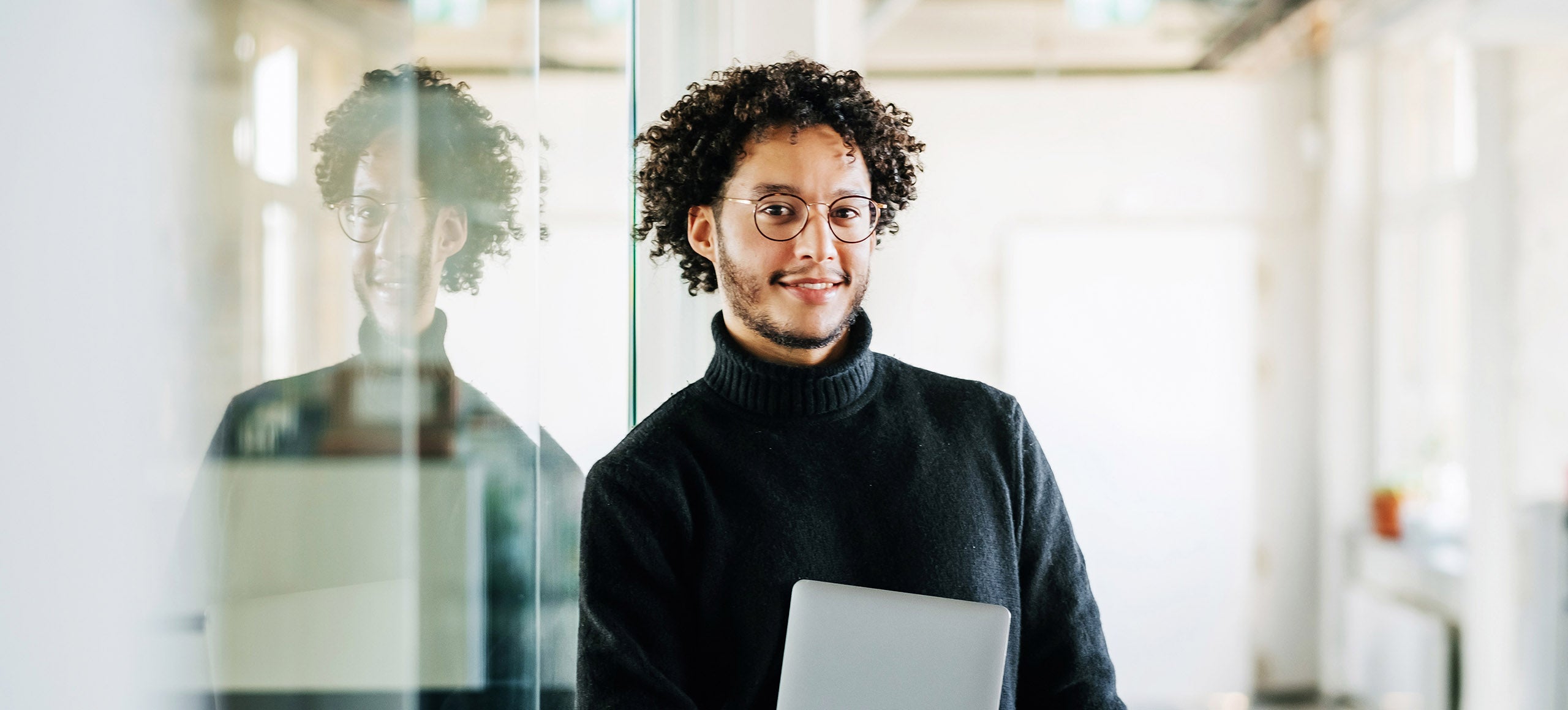
Ever heard of cluster analysis? If not, you’re in for a treat.
As a powerful data-mining tool, cluster analysis can help your organisation to identify different customer groups, and their typical behaviours. But why is that helpful? And what can you use cluster analysis for in the context of market research?
In this complete guide, we’ll help you to understand what cluster analysis is, when to use it, and how it can help your business. We’ll also talk you through the cluster analysis process, cover different types of cluster analysis, and clear up how cluster analysis and factor analysis are different.
Let’s dive straight in.
What is cluster analysis?
Cluster analysis (otherwise known as clustering, segmentation analysis, or taxonomy analysis) is a statistical approach to grouping items – or people – into clusters, or categories.
The objective of cluster analysis is to sort subjects into groups based on similarities: if there’s a high degree of association, subjects would be placed into the same group. Conversely, a low degree of association would see subjects placed in different groups.
Cluster analysis is unique as a statistical method, in that it’s conducted without the foundation of an assumed principle or fact; instead, this type of analysis is primarily concerned with data matrices where variables haven’t yet been split into criterion vs. predictor subsets.
Wow, that was technical.
Still with us? Great.
Because cluster analysis is what’s known as an ‘unsupervised learning algorithm’, you won’t know how many different clusters you’re dealing with beforehand. In fact, this approach is specifically employed when no assumptions have previously been made about expected relationships within the data you’re studying.
Cluster analysis will give you insight into where patterns and associations may be present within specific data, but it won’t interpret what those associations are, or what they may mean.
How can cluster analysis be used?
Cluster analysis can be used to great effect in market research. Most commonly, cluster analysis is concerned with classification: in other words, arranging subjects into different groups based on certain similarities. The goal of classification is that subjects in the same group would be more like one another than to subjects in a different group.
In the context of market research, this is particularly helpful for splitting people into any number of useful categories – such as location, age bracket, earning potential, education level, and even buying behaviours.
For marketers, cluster analysis is invaluable for audience segmentation as it makes it possible to target specific groups of customers with relevant, tailored messaging – increasing the chances of creating a connection and eliciting a response from your intended audience.
From a public health perspective – and most notably seen throughout the Covid-19 crisis – cluster analysis can even be used by healthcare researchers to identify locations with particularly high (or low) levels of illness.
No matter what use cluster analysis is put to, it is invaluable for market research – where knowledge truly is power. In fact, we’re such big believers in the need to map out your market that we’ve developed industry-leading software to help you on your way.
Forsta’s market research survey software allows you to investigate your market (from target audience to top competitors), analyse the data, and act on the findings. Find out more.
Cluster Analysis Process
So, what’s the cluster analysis process all about?
Three simple steps.
When you’re using cluster analysis to find out more about your target audience ahead of a big product launch or design iteration, it’s all about getting down to the nitty gritty of how they’re behaving, and what’s making them tick.
And this is how you do it.
Step one: create your survey
First things first, you need to build – and distribute – a survey. But what sort of survey, we hear you ask? Well, it should be designed to incorporate different measures of customer preference for your product, how likely they are to buy it, and the factors that could influence their decision. You need to send your survey to a decent sample size of your target customers, as if the sample size is too small, you won’t be able to elicit enough data to make statistically informed decisions.
Step two: analyse your findings
The next step is to reduce your data with a factor analysis of your survey (this minimises the factors that are being clustered by identifying multiple questions monitoring the same thing – allowing you to combine them before cluster analysis takes place). Once that’s done, you’ll be able to carry out a cluster analysis, figure out the right number of clusters, and get grouping!
Step three: act on your findings
When you have your clusters, you can view data across these different groupings (and name them according to their differences). It’s these essential differences that will allow you to tailor and target your marketing and advertising efforts according to specific groups of customers.
Types of cluster analysis
When it comes to choosing which type of cluster analysis to perform, you have three key methods to pick from: hierarchical cluster, K-means cluster, and the two-step cluster (which sounds a little like a dance, right?)
Let’s look at what each method brings to the table.
Hierarchical Cluster
Hierarchical clustering (the most common approach, if you’re asking), groups together variables in a way that’s reminiscent of factor analysis. It begins by treating every observation as a separate cluster, before repeatedly identifying the two clusters that are most similar, and then merging them. This continues until all clusters are merged – creating a set of clusters, with each cluster distinct from the other. Hierarchical cluster analysis can work with nominal, ordinal, and scale data – so long as you don’t mix in different levels of measurement.
K-Means Cluster
The K-means cluster comes into its own when you need to quickly cluster large sets of data. With this method, researchers define how many clusters there’ll be before carrying out the study. In fact, The K in ‘K-means’ stands for the number of clusters you’re trying to identify.
Two-Step Cluster
This best-of-both-worlds approach combines hierarchical and K-means clustering – automatically selecting the number of clusters. By carrying out pre-clustering first followed by hierarchical clustering, two-step clustering uses a cluster algorithm to identify different groups. This method is great for large datasets that hierarchical clustering would take too long to process.
When to use cluster analysis
Now that you understand a little more about the nature of cluster analysis, let’s look at when you ought to use it.
Cluster analysis is most often carried out during the initial, exploratory phase of research to uncover different structures in data. It doesn’t provide an explanation or interpretation of that data, but instead identifies specific groups within a population – without explaining why those structures exist the way they do. But that’s okay!
Cluster analysis is an important part of market research, as it presents different groupings for analysis. Once these groups have been defined, you can use the data surrounding each cluster to understand certain things about your target market: are they likely to buy your product? What sort of messaging will they respond to? What form of comms do they favour?
For that reason, cluster analysis is especially useful when you’re planning to launch a new product, update an old one, or rollout a marketing campaign. The ability to target potential customers in a focused, informed way truly is invaluable here. You can even use cluster analysis to create specific offers or products that are tailored to one particular group. Clever stuff!
Cluster analysis vs factor analysis?
Right then, what’s the big difference between cluster analysis and factor analysis?
We’ve already touched on this above, but factor analysis is basically a way of reducing large numbers of variables by removing overlapping questions that relate to the same concept, leaving you with a more refined number of clusters.
You’d use factor analysis when tackling a particularly complex survey or fighting your way through an inordinate number of variables. But rather than using factor analysis in place of cluster analysis, it’s best to use it beforehand – simplifying your data so that it’s easier to process and find true patterns.
Ultimately, the objectives of cluster analysis and factor analysis are different: cluster analysis is intended to divide observations into distinct and homogenous groups, while factor analysis is intended to explain the homogeneity of variables that result from similar values. Different, see?
Ready to get your cluster on?
Cluster analysis is a fantastic way of understanding the different groups of your target audience – and for determining if they’re your target audience at all.
At Forsta, we firmly believe that the more you know about your customers, the more powerful your product will be. So, let’s get clustering.
Related stories
Boost your agency’s performance: 5 AI strategies for success
Boost your agency’s performance: 5 AI strategies for success Webinar synopsis: AI is revolutionizing research, delivering faster, more accurate insights. This webinar covers five key strategies to integrate AI into your agency, enhancing efficiency, decision-making, and client results—while preserving the human touch. Through case studies and expert insights, you’ll leave with actionable steps to overcome […]

Power up your profit
Power up your profit: Elevating financial results with operational excellence Power up your profit: Elevating financial results with operational excellence Operational excellence is your key to retail success. Learn to build a cost-effective customer acquisition model, use insights for real results, and leverage employee experience. Enhance local operations and deliver exceptional customer experiences. With actionable […]

Human-centered design for market research
Human-centered design for market research Human-centered design for market research Dive into the world of human-centered design (HCD) in market research with Forsta The secret to outstanding insights starts with the people you’re asking. We’re here to share how empathy drives actionable insights, holistic data analysis accelerates understanding, and compelling storytelling enhances engagement. At Forsta, […]

Learn more about our industry leading platform
FORSTA NEWSLETTER
Get industry insights that matter,
delivered direct to your inbox
We collect this information to send you free content, offers, and product updates. Visit our recently updated privacy policy for details on how we protect and manage your submitted data.